Antibiotic resistance: discovery of a million antimicrobial peptides
With the rise of antibiotic resistance, the discovery of new antibiotic molecules has become an urgent priority. Nearly a million potential antimicrobial peptides have been identified with machine learning. ¹
Sources
This article is based on scientific information
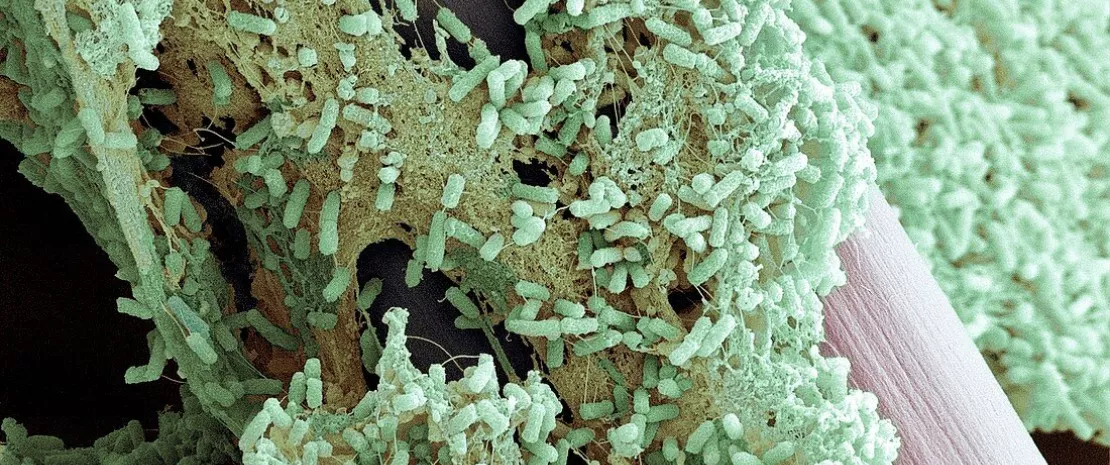
About this article
Finding new antibiotics to fight resistance is an urgent challenge facing medicine and (sidenote: Machine Learning Automatic learning whereby artificial intelligence solves a task based on metagenomic and metabolomic data collected, in this case the identification of discriminating bacterial species. Wazid M, Das AK, Chamola V, et al. Uniting cyber security and machine learning: Advantages, challenges and future research. ICT Express, 2022; 8(3), 313-321. ) may offer a helping hand. How? By predicting the global reservoir of (sidenote: Antimicrobial peptides (AMPs) Antimicrobial peptides (AMPs) are short sequences of amino acids widely present in a variety of organisms, including bacteria, plants, amphibians, insects, fish, and mammals. They are capable of disrupting microbial growth, most often by interfering with cell wall integrity. Explore https://www.sciencedirect.com/topics/agricultural-and-biological-sciences/antim… ) present on Earth. These AMPs represent a promising therapy which has already been applied clinically in the form of antiviral drugs (e.g. enfuvirtide). They are currently undergoing clinical trials for immunomodulatory properties that can treat microbial infections (yeast, bacteria). Their advantages over antibiotics are considerable: some have a narrow spectrum, thus enabling more targeted therapies, while resistance to many AMPs evolves slowly and is not linked to cross-resistance with other widely used classes of antibiotics.
1.27 million Antibiotic-resistant infections currently kill 1.27 million people per year. ¹
Nearly a million candidates
In practice, researchers have used machine learning-based methods to predict and catalog candidate AMPs (c_AMPs) produced by all terrestrial organisms by sifting through global public databases (63,410 metagenomes and 87,920 bacterial or archaeal genomes). The authors restricted themselves to a particular category of AMPs, those encoded by the organisms’ own genes, amounting to fewer than 100 amino acids. This resulted in the creation of the AMPSphere, a collection of 863,498 non-redundant peptide sequences.
The majority (91.5%) of these c_AMP sequences had not been previously described. The AMPSphere spans 72 different habitats, which were classified into eight high-level habitat groups (soil/plant: 36.6%; aquatic: 24.8%; human gut: 13%, etc.). Most of these c_AMPs are rare and habitat specific. The majority come from the microbial world, and four of the five bacterial genera that provided the most c_AMPs to the AMPSphere are found in a host (three of which – Prevotella, Faecalibacterium, and CAG-110 – are commonly found in animals).
100 candidates successfully tested
To validate the anti-microbial power of c_AMPs, the researchers synthesized 100 of them and tested them against resistant pathogenic bacteria and commensal bacteria of the digestive system. In vitro, 79 were active against pathogens or commensals, including 63 against 11 pathogens recognized as public health concerns. Four peptides were effective (100% of cells killed after 24 hours incubation at 37°C) at very low concentrations of around 1 µmol/L, comparable to the most potent peptides described in the literature.
Microbiota at the forefront of antibiotic resistance
What are the mechanisms of action? Studies of peptides active against Acinetobacter baumannii or Pseudomonas aeruginosa show that they significantly permeabilize the pathogens’ outer membrane. The efficacy of the main candidate AMPs has also been confirmed in vivo in murine abscess models (infection by A. baumannii).
These in vitro and in vivo experiments demonstrate the ability of machine learning to identify functional AMPs from the global microbiome.